Hi folks! Xiao’an here, Musiio’s resident music nerd and strategist. I’ve composed music for games and commercials for some years before joining the team.
Part of my job is to shine a light on the black box that is the AI’s decision making process. We know it knows a lot - it’s listened to 2 centuries of music. But exactly “how” it makes those decisions is less of a straightforward question.
So we’re going to subject the AI to a test today by tagging the constituent parts of a song. We’ll take a look at how the tags of each part differ, and how this impacts the tags assigned to the complete song.Â
As human listeners, it’s easy to forget that we all have biases which affect our judgement - this might be memories of a piece of music, or just down to personal taste. Part of the power of AI is that it’s able to listen to the same piece of music with an unbiased view, every single time.
So let’s see what it tells us!
ROSANNA, by TOTO
Let’s listen to the full track.
https://youtu.be/qmOLtTGvsbM
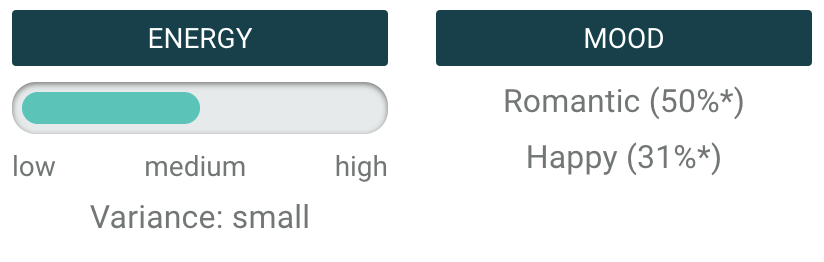
Now let’s hear it without drums:
http://youtube.com/watch?v=XwWqsrMQyfE
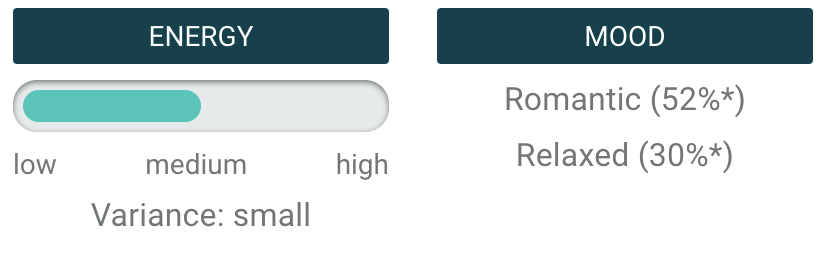
Now let’s hear the drums by themselves:
https://www.youtube.com/watch?v=DQ-gfb6nUus
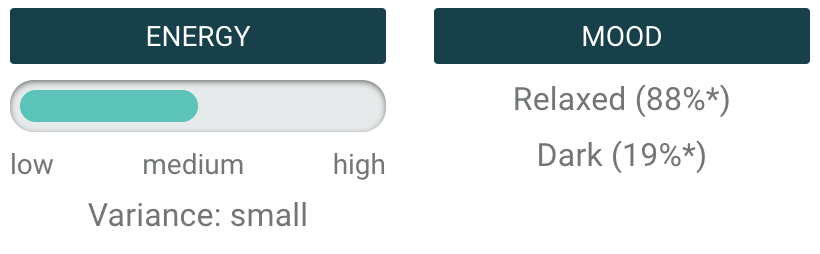
Each of these versions/constituents of the song sounds radically different, and we’ve analyzed them separately. While the parts have unique emotional identities, their combination yields surprising results. The Drum part was tagged Relaxed and Dark, and the Drumless version was tagged Romantic and Relaxed. In the full track, Romantic remains, Dark disappears, and Happy appears.
Therefore, the full track is not simply a sum of its parts. It is something new that is shaped by the relationships between its constituent parts. For example, a drum part may feel “powerful”, and a bass part may feel “relaxed”, but they may interact in such a way that a new feeling of “groovy” is created.
This points to the objectivity of the AI, which has no personal preferences. It analyzes an audio file as a whole, while a guitarist or drummer might be inclined to judge a piece of music more favorably or harshly based on the specific instrumental part. Perhaps it is a lesson to us that we should make professional judgments based on the whole product versus getting the type of tunnel vision that a highly technical viewpoint is vulnerable to.
Let’s take a look at another piece of music in the same way:
FIXER, by DAVID ORTEGA

A great track with clear Nine Inch Nails influences. I’m sure most people would agree with the AI’s assessment that it is powerful and dark, with a largely negative emotional valence. Now let’s check out the individual parts (also known as Stems).
Drum Stem

Bass Stem

Guitar Stem

Synth Stem

There are a lot of related Moods in the Stems. Let’s group some of the most interesting ones into a few related categories that are also observed in the Full track:
Energetic, Powerful - High energy/impact
Dark, Dramatic, Scary, Angry - Ominous or negative-skewing
In a way, these Stems are fairly consistent in mood with the Full track in that they contain either qualities associated with “Powerful” or with “Dark”. However, from the emotional range displayed, we can see that the final track is not one-dimensional in this regard. Rather, its Moods are a complex and nuanced combination of a variety of colors.
Let’s look at Dark, for example. Dark is not always Scary, but perhaps the Synth stem lends some of that color to this track. Dark is not always Angry, but perhaps the Guitar stem adds a bit of that specific emotion.Â
Want to chat about AI? Get in touch with us here!